十七作文
“dontbeyourself”通过精心收集,向本站投稿了18篇十七作文,下面是小编为大家整理后的十七作文,仅供参考,喜欢可以收藏与分享哟!
篇1:十七
这个夏天,似乎格外炎热,是想为我留下对十七岁深刻的记忆吗?
走在十七岁的尾巴上,不禁对过去产生怀念。童年的快乐时光已经过去,离开了的,就再也不可能回来了。
时间,永远不可能回到最开始的地方。但时间越是向前,我越对过去怀念。
十七岁,一个如花绽放的年龄,充斥着太多快乐、辛酸和令人无法忘怀的事情。
依稀记得,自己曾经在空间里收藏了一组叫《那年我们十七岁》的图片。看着那组图发觉好多事情都在十七岁悄然发生着。
对未来的憧憬,对友情的珍惜,对爱情的懵懂,对亲情的叛逆……
十七岁,人生中最后一个单纯的岁月。
这个年龄的人,性格倔强。但终究还只是个孩子,仍然有些许的稚气。
幻想天长地久,期待不离不弃,渴望一起长大。
你是在等待某个人的出现吗,还是为了实现对某人的承诺?
十七岁,有无助,有分离,有选择,有背叛……
有时,生活像一杯白开水,无色无味,令人乏味。
有时,生活像黑白老照片,简单枯燥,毫无生气。
我的方向在哪,我该何去何从?
风筝断线,虽然脱离了束缚,从此自由自在,但是最终也要坠向地面。
时间回不到最开始的地方。
但故事尚未结束,有许多故事在十七岁才刚刚开始。
也许,在这时,生命中出现了一个对自己很重要的人。
我们应该珍惜这个人,用真心,用关爱。
我们应该看到,十七岁的天空,永远蔚蓝,十七岁的笑容,永远纯真。
篇2:十七
时间,总是那样匆匆忙忙,如同一辆载着生命的列车,匆忙地将他们送去下一个年龄的驿站。十六岁的生活就这样浑浑噩噩的过完了,静静得迈入了十七岁的门槛,感觉过得很快,也变得很快,曾经的轻狂变成了现在的稳重,轻率变成了沉着,无忧变成了郁闷……浑浑噩噩中发生了冥冥之中注定但又不知不觉的改变。
回头看看自己走过的路,才发现那是一片苍茫,于是此时才明白,原来成长不过是血淋淋地正视自己的寂寞和对未来路途的迷惘。
记得幼时听人说十七岁是雨季,于是很向往,可那时却怎知这样的季节里掉落的都是青涩少年苦涩的泪水。
十七岁,知道了心痛的滋味。努力了一年,当那惨烈的分数映入眼帘,会听到有什么碎掉的声音,那是心碎的声音,是明明努力了却得不到酬偿的悲痛。鼻子酸酸的,眼眶中瞬间积满了晶莹的液体,但那点可怜的自尊与固执却逼迫自己忍住了泪水。
十七岁,常常会被感动,为一件微不足道的小事,为小说主人公悲惨的命运而哭得稀里哗啦,只因为它触动了心底那块最柔软的地方。
原以为,十七岁的我应该更成熟了吧,更加坚强,更加自信,可当自己一天天沉陷在雨季的沼泽里,才明了这是命中注定的不可缺少的经历。
有人说,成长就是习惯孤独,习惯挫折。习惯了就不会再哭了吧。
我会试着慢慢习惯,我会努力不让自己的十七岁变成雨季,我会不断告诫自己:十七岁不哭。
篇3:十七
十七岁,意味着什么?是情窦初开的年龄,是迈向十八的大门,是努力拼搏的青春。
十七岁那年的雨季,我们有着共同的期许,也曾经紧紧地拥抱在一起。正如歌声中那般,十七岁的我们有着天潭般的幻想与不切实际的目标。即便如此,我们还是不知天高地厚地去追求,去尝试。因为,十七岁的我们始终相信:只要用尽全力地去拼搏,梦想终会开花结果。
也许,十七岁的我们在追求梦想的道路上有着许多迷茫,甚至一度地想去放弃。当时的我们或许是累了,或许是空手而归,便想着半途而废。可这样做,我们真的心甘情愿放弃之前的所有努力吗?拼搏了那么久,离成功只差一步DD高考。高考相当于战场,决定着我们的未来的道路。无论这一步多么艰难,也应该咬着牙走过去,坚持到最后。因为,走过去的你会发现:你很感谢当初坚持拼搏的自己,才能有现在如此成就。
十七岁的我们,会异常的敏感与脆弱,对外界的所有一切都有着防御之心。那是因为我们害怕失去,害怕面对,害怕最后只剩路灯照下的身影。曾有人对我说过:世间最可怕的不是流言蜚语,而是自己的敏感与脆弱。众所周知,流言蜚语可以扭曲事实的真实性,甚至能够侵犯一个人的清白,可见是何等的可怕。然而,敏感与脆弱往往能够使自己误入歧途。其实这都源自于自身承受不住外界所带来的压力,把事情想像的复杂化而造成的。所以,十七岁的我们要笑口常开、敞开心扉,勇敢去面对以后所走的每一步。
十七岁的我们,正迈向着十八的大门,在离成年的距离越来越近,即将面临着社会的严峻考验。当面对着层出不穷的诱惑时,必须要有强大的自律能力去抵抗。不要因一次的好奇心去尝试而误入歧途,那么最终的你将会后悔不已。同时在面对社会所带来的重重关卡时,我们只有在现在去努力拼搏,巩固强大的基础。这样,十七岁的我们才能看到前方胜利的曙光。
十七岁,很浪漫,很美好!愿十七岁的我们永不忘初心勇敢地走下去。
篇4:十七
无尽的思绪让我执起笔来,写下这无限的心情交响乐,不容错失。某某人曾说过:不是我想写它,是它来找我去写。此刻,我似乎与之产生了共鸣。
世人常叹时间的无情飞逝。的确如此。窥镜自视,昔日……如今……无言的痕迹。话破了时光的掌纹,深深,烙印。璀璨的年华早已悄然溜出了手掌,五指,抓不住,挽不回。十七,告别我的青春年华。我企望,我盼望,我渴望,十七岁的日子可以增长、增长、再增长吗?一恍惚、一眨眼,一个春冬又悄然偷世。为什么?世人都喜欢问为什么!轮回依然在转动,无穷尽。为了又会怎样?不问又会怎样?知道后又会怎样?
看着别人在学,自己也决心发奋,当那人消失了,什么决心不决心的,早已忘记得一甘二净了。人,始终是善变的动物!高二了,总不能不学。无奈,日子如梭。有人苦叹日子如年,我却伤感日子似水。几度云卷云舒,几许花开花落,人间不断地循环循环。开始即是结束,结束亦是另一个开始。世界的一切想必也遵循这一个规律运转,一直、不断、运转……学那么多又有什么用呢?人总该赤裸裸地去。但,现实的残酷证明了:我不得不学。水向低处流,人向高处爬,这又是自然的规律,无法解析。
总想,在十七的日子里留下些什么,将来能可回味、纪念。纪念我死去的青春,纪念岁月车轮辗过的声息,纪念十七的一切一切……我搜索着脑瓜,是否有这么一个人,站在来路,也站在去路,让我牵挂得肝肠寸断。依稀,梦里的人儿,你在何处?徘徊,伫足,凝眸。细碎的阳光覆盖着明亮的黑睛,看不清。是你吗,疼我的人儿?
年年岁岁淡淡,时光扔下我轰轰烈烈地向前奔跑。青春,再见了。狂妄的日子,再见了。十七岁的夏天,将又会是怎样的季节呢?我带上微笑开始了新的旅程……
篇5:十七作文
十七作文
时间一天天的过去,又一天天到来,就像流水一样,来来去去,川流不息。
站在岁月的风中,轻轻的对着窗前的风铃说:“我已十七岁,勇敢的走过了十七个春夏秋冬”。在酒满欢笑和泪水的人生道路上,蓦然回首,才发现原来所走过的路就像一道绚丽的彩虹,历经风雨的洗礼。曾经的烦恼和痛苦都烟消云散,变得很谈,很谈,谈得就像过烟云烟。
也许:
日子过的太匆忙,让我忽视了岁月的流逝。
也许
由于生命过得太沉重,使我忘记了季节的'更替。
但,这一切,我都毫不在乎。因为,我懂得,生命的真谛在于奋斗。也只有奋斗,才能让人生的沃土上开满鲜花,无比灿烂。
到了十七岁:多了一份成熟,少一份天真:多了一些追求,少了一些幻想:多了一些微笑,少了一些泪水:多了。我对着天空骄傲地说:“这就是我的十七岁”,对未来充满希望的十七岁”。
人生,就如同一张画板,我不知十七岁该在上面涂上什么颜色,但我决不会让它一片空白。也许,我会涂得不太好看:也许,我会涂得太谈:也许,我会涂爹太艳:但,我想:有颜色的青春总会比没有颜色的青春变多
姿多彩一些和更有活力。
十七岁,我开始有了新的追求。在这世间,美好的东西实在数不过来,总希望能得到太多,而害怕失去,而往往失去很多。所以:我就追求得很简单,也很直得,就是在每分每秒中把握着摸测的未来和梦。
十七岁,你是我人生中的一道美丽的风景线。我一定会在人生的画板上染上你的应有的颜色。
篇6:十七、PythonSQLAlchemy
If you‘re using SQLAlchemy version 0.3, don‘t worry ― everything you‘ll learn in this tutorial still works just fine under SQLAlchemy 0.3. Nearly all the changes to SQLAlchemy between 0.2 and 0.3 were either internal code cleanups, or else were changes to advanced features that this tutorial doesn‘t cover. Therefore, I probably won‘t give this tutorial a rewrite for SQLAlchemy 0.3.
Now, on with the tutorial.
篇7:十七、PythonSQLAlchemy
让我们首先从一个简单的SQLALchemy程序开始。复制如下的内容并且命名为firststeps.py.
from sqlalchemy import *db = create_engine(‘sqlite:///tutorial.db‘)db.echo = False # Try changing this to True and see what happensmetadata = BoundMetaData(db)users = Table(‘users‘, metadata, Column(‘user_id‘, Integer, primary_key=True), Column(‘name‘, String(40)), Column(‘age‘, Integer), Column(‘password‘, String),)users.createi = users.insert()i.execute(name=‘Mary‘, age=30, password=‘secret‘)i.execute({‘name‘: ‘John‘, ‘age‘: 42}, {‘name‘: ‘Susan‘, ‘age‘: 57}, {‘name‘: ‘Carl‘, ‘age‘: 33})s = users.select()rs = s.execute()row = rs.fetchone()print ‘Id:‘, row[0]print ‘Name:‘, row[‘name‘]print ‘Age:‘, row.ageprint ‘Password:‘, row[users.c.password]for row in rs: print row.name, ‘is‘, row.age, ‘years old‘
篇8:十七、PythonSQLAlchemy
This code sample shows off a lot of the features of SQLAlchemy. Let‘s go through it step-by-step.上面的代码涉及到了SQLAlchemy的很多特性,让我们来详细介绍一下。
from sqlalchemy import *db = create_engine(‘sqlite:///tutorial.db‘)
The first step in writing SQLAlchemy code is to open a connection to the database you‘ll be using. In SQLAlchemy, this is done by creating an SQLEngine object, which knows how to talk to one particular type of database (SQLite, PostgreSQL, Firebird, MySQL, Oracle...). The SQLEngine object also doubles as a connection object. Behind the scenes, it will create a pool of database connections and re-use them automatically as needed, to keep your application running quickly. But most of the time, you don‘t even need to think about the database connections; just use theSQLEngine object that create_engine() returns, and let SQLAlchemy handle the details for you.
New in 0.2: In SQLAlchemy 0.1, the syntax of create_engine() calls would depend on which database engine you were using, and wasn‘t very userfriendly. Now it‘s much more consistent. No matter what database you‘re using, the create_engine() function takes a single parameter that‘s a URI, of the form. “engine://user:password@host:port/database”. Most of these options can be omitted; for example, if you‘re connecting to a PostgreSQL database on the default PostgreSQL port (port 5432), the URI would be something like “postgres://scott:tiger@localhost/demodb”. SQLite lets you omit everything but the filename, so opening a file named tutorial.db in the current directory becomes “sqlite:///tutorial.db”. Or, to open the file “/tmp/tutorial/joindemo.db”, the URI becomes “sqlite:////tmp/tutorial/joindemo.db”. (Yes, that‘s four slashes in a row. Two before the (empty) hostname section, then one before the database section, and one final slash at the start of the path “/tmp/tutorial/joindemo.db”.)
metadata = BoundMetaData(db)
Before creating our Table definitions, we need to create the object that will manage them. Table definitions (what the columns are called, what their data types are) are an example of “metadata” -- information about your data. So the object that manages this collection of metadata is called a MetaData object. There are two varietes, BoundMetaData which is tied to a specific database connection, or DynamicMetaData which can be created before the database connection has been established.
If you don‘t know why you‘d want to use DynamicMetaData, or if you didn‘t really understand the previous paragraph, don‘t worry about it. Just remember that you‘ll need a BoundMetaData to keep your Table objects in, and move on to the rest of the tutorial.
New in 0.2: In SQLAlchemy 0.1, you‘d pass the db object to your Tables; and, in fact, this is still allowed; it will automatically create a BoundMetaData object for you. It‘s better to create theBoundMetaData object explicitly, though, because that makes it a lot easier to do operations like metadata.create_all() to create all your tables at once.
users = Table(‘users‘, metadata, Column(‘user_id‘, Integer, primary_key=True), Column(‘name‘, String(40)), Column(‘age‘, Integer), Column(‘password‘, String),)users.create()
This should be pretty self-explanatory: we‘ve just created a users table in our database, with four columns. If the users table already existed, we could instead have done:
users = Table(‘users‘, metadata, autoload=True)
and SQLAlchemy would have automatically figured out the table‘s structure from the database.
i = users.insert()i.execute(name=‘Mary‘, age=30, password=‘secret‘)i.execute({‘name‘: ‘John‘, ‘age‘: 42}, {‘name‘: ‘Susan‘, ‘age‘: 57}, {‘name‘: ‘Carl‘, ‘age‘: 33})
SQLAlchemy‘s SQL construction methods are beautiful. You‘ll almost never need to write SQL by hand. Instead, you create an “SQL statement object”, build the SQL query you want, and call itsexecute() method. Here we ask for an INSERT statement referencing the users table:
i = users.insert()
Now when we do i.execute(), SQLAlchemy will generate the appropriate “INSERT INTO users VALUES (...)” statement for the values we pass into execute(). Notice the two different ways of executing an INSERT statement. We can either pass it keyword parameters, to insert a single object:
i.execute(name=‘Mary‘, age=30, password=‘secret‘)
or else we can pass it multiple dictionaries, to insert multiple objects:
i.execute({‘name‘: ‘John‘, ‘age‘: 42}, {‘name‘: ‘Susan‘, ‘age‘: 57}, {‘name‘: ‘Carl‘, ‘age‘: 33})
If you have any “special” characters (such as semicolons or apostrophes) in your data, they will be automatically quoted for you by the SQLEngine object, so you don‘t have to worry about quoting. This also means that unless you deliberately bypass SQLAlchemy‘s quoting mechanisms, SQL-injection attacks are basically impossible.
You may have also noticed that we didn‘t have to specify all the columns of the database. Any columns we didn‘t specify will get filled with NULL, except for the primary key, which will automatically get a unique value.
s = users.select()rs = s.execute()
Like INSERT statements, SELECT statements are also done by creating a statement object and calling its execute() method. This particular statement is the basic “SELECT * FROM users” with noWHERE clause. Later on we‘ll see how to do WHERE clauses, or only return data from certain columns, or JOIN two (or more) tables together.
Calling execute() on a SELECT statement object will return a result set, which has fetchone() and fetchall() methods. As you‘d expect, fetchone() returns a single row, while fetchall() returns a list of rows. The rows returned aren‘t simple tuples or dictionaries, but intelligent row objects, as can be seen below:
row = rs.fetchone()print ‘Id:‘, row[0]print ‘Name:‘, row[‘name‘]print ‘Age:‘, row.ageprint ‘Password:‘, row[users.c.password]
Here we see some of the various ways you can access the data in a row object. First, you can pretend it‘s a tuple and access its columns by position. SQLAlchemy guarantees that the column order returned from a “SELECT * FROM (table)” statement will be the same as the order in which the columns were declared in that table, so here we know that row[0] will be the user_id column. We can also access the row as if it were a dictionary (row[‘name‘]). Next, my favorite: SQLAlchemy lets us access the columns as if they were attributes of the row object. (Some simple __getattr__() magic behind the scenes makes this work). And finally, we can even use the actual Column objects themselves as keys to lookup results from a row. You probably won‘t use this very often, but it can be extremely useful in some circumstances.
for row in rs: print row.name, ‘is‘, row.age, ‘years old‘
Finally, we see that we can also iterate through the result set via a simple for loop. This is especially useful when you expect your SELECT query to return a huge result set that would be too large to load into memory: the for loop will only fetch one row at a time from the database.
篇9:十七、PythonSQLAlchemy
Now let‘s take a little time to examine some of the various ways in which we can select rows from our database. There are lots of conditions you might want to put in the WHERE clause of a SELECTstatement, and SQLAlchemy makes most of those easy.
Copy the following code into “selectdemo.py”:
from sqlalchemy import *# Let‘s re-use the same database as beforedb = create_engine(‘sqlite:///tutorial.db‘)db.echo = True # We want to see the SQL we‘re creatingmetadata = BoundMetaData(db)# The users table already exists, so no need to redefine it. Just# load it from the database using the “autoload” feature.users = Table(‘users‘, metadata, autoload=True)def run(stmt): rs = stmt.execute for row in rs: print row# Most WHERE clauses can be constructed via normal comparisonss = users.select(users.c.name == ‘John‘)run(s)s = users.select(users.c.age < 40)run(s)# Python keywords like “and”, “or”, and “not” can‘t be overloaded, so# SQLAlchemy uses functions insteads = users.select(and_(users.c.age < 40, users.c.name != ‘Mary‘))run(s)s = users.select(or_(users.c.age < 40, users.c.name != ‘Mary‘))run(s)s = users.select(not_(users.c.name == ‘Susan‘))run(s)# Or you could use &, | and ~ -- but watch out for priority!s = users.select((users.c.age < 40) & (users.c.name != ‘Mary‘))run(s)s = users.select((users.c.age < 40) | (users.c.name != ‘Mary‘))run(s)s = users.select(~(users.c.name == ‘Susan‘))run(s)# There‘s other functions too, such as “like”, “startswith”, “endswith”s = users.select(users.c.name.startswith(‘M‘))run(s)s = users.select(users.c.name.like(‘%a%‘))run(s)s = users.select(users.c.name.endswith(‘n‘))run(s)# The “in” and “between” operations are also availables = users.select(users.c.age.between(30,39))run(s)# Extra underscore after “in” to avoid conflict with Python keywords = users.select(users.c.name.in_(‘Mary‘, ‘Susan‘))run(s)# If you want to call an SQL function, use “func”s = users.select(func.substr(users.c.name, 2, 1) == ‘a‘)run(s)# You don‘t have to call select() on a table; it‘s got a bare forms = select([users], users.c.name != ‘Carl‘)run(s)s = select([users.c.name, users.c.age], users.c.name != ‘Carl‘)run(s)# This can be handy for things like count()s = select([func.count(users.c.user_id)])run(s)# Here‘s how to do count(*)s = select([func.count(“*”)], from_obj=[users])run(s)
Most of this should be pretty self-explanatory, and should give you an idea of what‘s possible. A few notes:
# Or you could use &, | and ~ -- but watch out for priority!s = users.select((users.c.age < 40) & (users.c.name != ‘Mary‘))run(s)s = users.select((users.c.age < 40) | (users.c.name != ‘Mary‘))run(s)s = users.select(~(users.c.name == ‘Susan‘))run(s)
In Python, the & (and), | (or), and ~ (not) operators have a higher priority than comparison operators like == and !=. So if you want to use the &, | and ~ operators, you have to be careful to wrap the other clauses in parentheses. If you forget the parentheses, you‘ll be surprised by the results:
s = users.select(users.c.age < 40 & users.c.name != ‘Mary‘)
will be interpreted as:
s = users.select(users.c.age < (40 & users.c.name) != ‘Mary‘)
which will almost certainly not return the results you were expecting.
Finally, let‘s take a little bit of a closer look at the bare form. of select():
# You don‘t have to call select() on a table; it‘s got a bare forms = select([users], users.c.name != ‘Carl‘)run(s)s = select([users.c.name, users.c.age], users.c.name != ‘Carl‘)run(s)
Remember that the first argument to the bare form. of select() is a list. If you forget and pass it a table, you‘ll get a TypeError complaining about iteration over a non-sequence.
# This can be handy for things like count()s = select([func.count(users.c.user_id)])run(s)
When there‘s a table involved in the select() call (e.g., when you‘re counting the occurrences of a single column, such as users.c.user_id), select() knows what to do. But if you‘re trying to do something like a COUNT(*), the Select object won‘t be able to guess which table you want to select from unless you explicitly pass the from_obj parameter, like so:
# Here‘s how to do count(*)s = select([func.count(“*”)], from_obj=[users])run(s)
Note that from_obj expects a list, just like the “what to select” parameter does. Here, too, if you forget and pass a bare table, you‘ll get a TypeError about iteration over a non-sequence.
篇10:十七、PythonSQLAlchemy
Now for the really interesting part: mapping your objects onto the database.
In other object-relational mappers such as SQLObject, the table definition also doubles as the class whose instances are rows of data from the table. SQLAlchemy, on the other hand, makes a strict distinction between the table definition and the data class. You first create the table definition, then create an (empty) class definition that will hold your data objects, and then create a mapper that will map that class onto the database. It‘s perhaps easier to show how this works than to explain it. Copy the following into “mapper1.py”:
from sqlalchemy import *db = create_engine(‘sqlite:///joindemo.db‘)db.echo = Truemetadata = BoundMetaData(db)users = Table(‘users‘, metadata, autoload=True)emails = Table(‘emails‘, metadata, autoload=True)# These are the empty classes that will become our data classesclass User(object): passclass Email(object): passusermapper = mapper(User, users)emailmapper = mapper(Email, emails)session = create_session()mary = session.query(User).selectfirst(users.c.name==‘Mary‘)mary.age += 1session.flush()fred = User()fred.name = ‘Fred‘fred.age = 37print “About to flush() without a save()...”session.flush() # Will *not* save Fred‘s data yetsession.save(fred)print “Just called save(). Now flush() will actually do something.”session.flush() # Now Fred‘s data will be savedsession.delete(fred)session.flush()
Let‘s break this down piece-by-piece to see what‘s going on here.
from sqlalchemy import *db = create_engine(‘sqlite:///joindemo.db‘)db.echo = Truemetadata = BoundMetaData(db)
Here we‘re using the same SQLite database that we created in the join demo, which contains users and email addresses. Unless you‘ve deleted that file, the data (four users and four email addresses) should still be there as well. We set echo to True so that the SQL will be printed out at each step.
users = Table(‘users‘, metadata, autoload=True)emails = Table(‘emails‘, metadata, autoload=True)
Because the users and emails tables are already in the database, we don‘t have to specify them again; we can just let SQLAlchemy fetch their definitions from the database.
# These are the empty classes that will become our data classesclass User(object): passclass Email(object): pass
Note that your data classesmustbe new-style. classes (e.g., derived from the base class object). If they aren‘t, SQLAlchemy will raise an ArgumentError exception when you try to create the mapper. If you don‘t know what the difference between new-style. classes and old-style. classes is, don‘t worry about it; just get in the habit of deriving all your classes either from object or from another base class that descends from object. Most of Python‘s object-oriented features work much better on classes that ultimately derive from object, and SQLAlchemy makes heavy use of those features.
Moving on:
usermapper = mapper(User, users)emailmapper = mapper(Email, emails)
This is where all the magic happens. The mapper() function takes a minimum of two parameters: first the data class to modify, and then the table object onto which that data class should be mapped. The data class will have attributes automatically added to it that correspond to the columns of your database table. Thus, the User class now has User.user_id, User.name, User.age, andUser.password. The Email class now has Email.email_id, Email.address, and Email.user_id.
session = create_session()
SQLAlchemy is capable of automatically keeping track of the data objects you create, and any changes you make to their attributes. This is done using Session objects, which do a lot of the behind-the-scenes work for you. Usually, you‘ll only need one Session object. SQLAlchemy could create it for you, but there are times when you‘ll want to be able to access the Session object directly. So SQLAlchemy follows the Python principle that “Explicit is better than implicit” and requires you to create your own Session object. At least, that‘s the default behavior. there are advanced features that let you have a “default Session context”, and sometimes that‘s exactly what you want. However, it‘s best to learn the explicit way of doing things first; learning the advanced features can wait until you‘re comfortable with SQLAlchemy‘s way of doing things.
New in 0.2: Session objects replace the objectstore object from SQLAlchemy 0.1. The objectstore.commit() method (whose name was confusingly similar to the commit() method of database transactions) has been replaced by session.flush(). Session objects also have a couple of other differences, which we‘ll get to in a moment.
mary = session.query(User).selectfirst(users.c.name==‘Mary‘)
Now that we‘ve created a Session object, we can use it to load some data from our database. Now at first glance, the above line of code looks a little overcomplicated. You might be wondering why it isn‘t something simpler, like “session.selectfirst(users.c.name==‘Mary‘)”. The answer is that SQLAlchemy needs to know what class you‘re trying to create instances of. Here it might be possible to guess based on the fact that the selectfirst() criteria are specifying a column from the users table. But there‘s a Python principle that says, “In the face of ambiguity, refuse the temptation to guess.” Because what if that guess is wrong? What if you‘re trying to load all the email addresses belonging to people named Mary? By forcing you to be explicit about which data class you want, SQLAlchemy avoids the possibility of guessing wrong.
Incidentally, the session.query(YourClass) call creates a Query object that you could save and reuse later. This can save a lot of typing if you have multiple select() calls to make.
New in 0.2: In SQLAlchemy 0.1, you would have called selectfirst() on the usermapper object. In SQLAlchemy 0.2, select() (and all related functions such as selectfirst()) are called on Query objects instead.
mary.age += 1
Now that we have an instance of the data class (in the object “mary”), we can manipulate its attributes just like a normal object. SQLAlchemy will keep track of the changes we make, but won‘t actually send them to the database right away. To send our changes to the database, we need to ...
session.flush()
... call the session.flush() method. This will take all the changes we‘ve made to our data objects, and “flush” those changes out to the database. If we‘ve made multiple changes, some of which depend on other changes (e.g., adding a new user and several email addresses for that user), SQLAlchemy is smart enough to write the SQL statements in the correct order. SQLAlchemy also wraps the entire set of SQL statements in a database transaction, as you‘ll see if you examine the generated SQL code when you run this example.
If you want to control the database transactions yourself (maybe because you want to accumulate several calls to session.flush() before committing the transaction), you can. See the Transactions section, below, for details.
New in 0.2: The session.flush() function used to be called objectstore.commit(). The method name changed from commit() to flush() in 0.2 because commit() sounded like what you do to a database transaction, and it was causing some people to confuse SQLAlchemy sessions and database transactions, which are two completely separate concepts.
fred = User()fred.name = ‘Fred‘fred.age = 37
Here we‘re creating a new instance of our data class, User. This will eventually result in a new database row once we call session.flush().
print “About to flush() without a save()...”session.flush() # Will *not* save Fred‘s data yet
Look at the output from running this example. Notice that this session.flush() call resulted innodatabase activity whatsoever, even though we just created a new instance of our data class, which is supposed to automatically create a new row in our database table. Why didn‘t session.flush() do what it‘s supposed to do?
See if you can figure it out before proceeding to the next section. Hint: remember that you‘re not necessarily limited to having only one Session object around at any given time, and that “Explicit is better than implicit.”
session.save(fred)print “Just called save(). Now flush() will actually do something.”session.flush() # Now Fred‘s data will be saved
Now we see why the previous session.flush() call didn‘t actually save Fred‘s data to the database. The fred object wasn‘t yet associated with any Session objects! Explicit is better than implicit. By calling session.save(fred), we explicitly made session responsible for managing the fred instance. From now on, any changes made to the fred instance will be tracked by our session instance. That includes fred‘s current state of “instance has been created, but database row has not yet been created”. Any such “pending” objects will be flushed to the database at the next call to session.flush(), as you can see from the output of running the example.
As was mentioned before, there are advanced features that let you have a “default Session context”. If you‘re using those features, then any newly-created data instances (like our fred instance above) would be automatically registered with the Session object from the current context, and there‘d be no need to call session.save(fred) -- and therefore, the first session.flush() call in our above example would have created a new row in the database.
However, it‘s usually best to learn the explicit way of doing things first; learning the implicit way of doing things can wait until you‘re comfortable with the explicit way. Then, when you hit the limits of the implicit way and need to drop into “explicit mode” for a while, you won‘t be stepping outside the limits of your comfort zone. It‘s much harder to go the other way around: if you get too used to using implicit, default Session objects, then it will be hard to remember how to use them explicitly. This can lead to mistakes like calling flush() without calling save() first, followed by much head-scratching as you try to figure out why the database doesn‘t contain Fred‘s newly-created data.
New in 0.2: SQLAlchemy 0.1 used implicit Session objects, so calling objectstore.commit() after creating the fred instance would have run an INSERT SQL statement. In SQLAlchemy 0.2, you need an explicit session.save() call first, to associate the instance with one particular session. Read the above three paragaphs for more details.
session.delete(fred)session.flush()
Now that we‘ve looked at how to do INSERTs by creating new object instances, let‘s look at how to do DELETEs. DELETEs, like INSERTs, are done by calling a method on the Session object. Note that this does not delete the fred instance from your own code, it just flags it as “deleted” in the Session object‘s internal object tracker. The fred instance will still be around, accessible to your own code, until you run a “del fred” statement.
New in 0.2: In SQLAlchemy 0.1, the delete() method used to be called on the data class or on the mapper. In SQLAlchemy 0.2, you call delete() on the Session instance instead.
篇11:十七、PythonSQLAlchemy
If you want to control the database transactions yourself, instead of letting SQLAlchemy do it for you, you can obtain a Transaction object by calling session.create_transaction. You can then callcommit() or rollback() on the Transaction object. This allows you to safely do things like the following:
transaction = session.create_transaction()try: # Do some work here that might fail session.flush() # Do some more work here that might fail session.flush() # Success, commit everything transaction.commit()except: # Make sure the transaction is rolled back ... transaction.rollback() # ... then propagate the error upwards to be handled elsewhere raise
篇12:十七、PythonSQLAlchemy
At this point, you‘re probably wondering about using multiple tables in a single select() statement. Wonder no more. Copy the following into “joindemo.py”:
from sqlalchemy import *db = create_engine(‘sqlite:///joindemo.db‘)db.echo = Truemetadata = BoundMetaData(db)users = Table(‘users‘, metadata, Column(‘user_id‘, Integer, primary_key=True), Column(‘name‘, String(40)), Column(‘age‘, Integer),)users.create()emails = Table(‘emails‘, metadata, Column(‘email_id‘, Integer, primary_key=True), Column(‘address‘, String), Column(‘user_id‘, Integer, ForeignKey(‘users.user_id‘)),)emails.create()i = users.insert()i.execute( {‘name‘: ‘Mary‘, ‘age‘: 30}, {‘name‘: ‘John‘, ‘age‘: 42}, {‘name‘: ‘Susan‘, ‘age‘: 57}, {‘name‘: ‘Carl‘, ‘age‘: 33})i = emails.insert()i.execute( # There‘s a better way to do this, but we haven‘t gotten there yet {‘address‘: ‘mary@example.com‘, ‘user_id‘: 1}, {‘address‘: ‘john@nowhere.net‘, ‘user_id‘: 2}, {‘address‘: ‘john@example.org‘, ‘user_id‘: 2}, {‘address‘: ‘carl@nospam.net‘, ‘user_id‘: 4},)def run(stmt): rs = stmt.execute() for row in rs: print row# This will return more results than you are probably expecting.s = select([users, emails])run(s)# The reason is because you specified no WHERE clause, so a full join was# performed, which returns every possible combination of records from# tables A and B. With an appropriate WHERE clause, you‘ll get the# restricted record set you really wanted.s = select([users, emails], emails.c.user_id == users.c.user_id)run(s)# If you‘re interested in only a few columns, then specify them explicitlys = select([users.c.name, emails.c.address],emails.c.user_id == users.c.user_id)run(s)# There are also “smart” join objects that can figure out the correct join# conditions based on the tables‘ foreign keyss = join(users, emails).select()run(s)# If you want all the users, whether or not they have an email address,# then you want an “outer” join.s = outerjoin(users, emails).select()run(s)# Order of outer joins is important! Default is a “left outer join”, which# means “all records from the left-hand table, plus their corresponding# values from the right-hand table, if any”. Notice how this time, Susan‘s# name will *not* appear in the results.s = outerjoin(emails, users).select()run(s)
That‘s enough for a taste. More information can be found in the SQL Construction section of the SQLAlchemy documentation. Now let‘s move on to the really interesting part: mapping your data objects to SQL database rows.
篇13:十七、PythonSQLAlchemy
There‘s one more item to cover. We‘ve looked at one-to-many relationships, but we also need to look at many-to-many relationships. As you probably know already, many-to-many relationships in databases are handled by a third table that holds information about the relation. E.g., if you have an “articles” table and a “keywords” table, and you want to be able to associate keywords with articles, you‘d need a many-to-many relationship. One-to-many wouldn‘t work, because one article might need to be tagged with several different keywords, and the same keyword might be used to tag several articles; so this is a classic many-to-many relationship. Thus, you‘d use a third table with just two columns, “article_id” and “keyword_id”, to keep track of the associations. By convention, such a table is usually named with the names of the two tables it references, separated by an underscore. Thus, the table structure in the following example (call it “manytomany.py”):
from sqlalchemy import *db = create_engine(‘sqlite:///keywords.db‘)db.echo = Truemetadata = BoundMetaData(db)session = create_sessionarticles = Table(‘articles‘, metadata, Column(‘article_id‘, Integer, primary_key = True), Column(‘headline‘, String(150)), Column(‘body‘, String),)keywords = Table(‘keywords‘, metadata, Column(‘keyword_id‘, Integer, primary_key = True), Column(‘keyword_name‘, String(50)),)association = Table(‘articles_keywords‘, metadata, Column(‘keyword_id‘, Integer, ForeignKey(‘articles.article_id‘)), Column(‘article_id‘, Integer, ForeignKey(‘keywords.keyword_id‘)),)# Handy feature: create all the tables with one function callmetadata.create_all()class Article(object): def __init__(self, headline=None, body=None): self.headline = headline self.body = body def __repr__(self): return ‘Article %d: “%s”‘ % (self.article_id, self.headline)class Keyword(object): def __init__(self, name=None): self.keyword_name = name def __repr__(self): return self.keyword_name# To create a many-to-many relation, specify the association table as# the “secondary” keyword parameter to mapper()mapper(Article, articles)mapper(Keyword, keywords, properties = { ‘articles‘: relation(Article, secondary=association, backref=‘keywords‘),})a1 = Article(headline=“Python is cool!”, body=“(to be written)”)a2 = Article(headline=“SQLAlchemy Tutorial”, body=“You‘re reading it”)session.save(a1)session.save(a2)k_tutorial = Keyword(‘tutorial‘)k_cool = Keyword(‘cool‘)k_unfinished = Keyword(‘unfinished‘)a1.keywords.append(k_unfinished)k_cool.articles.append(a1)k_cool.articles.append(a2)# Or:k_cool.articles = [a1, a2] # This works as well!a2.keywords.append(k_tutorial)# Now we write all this out to the database in one single step, and# SQLAlchemy automatically figures out the correct order for the SQL# statements. Notice also how we didn‘t need to save the Keyword# instances, because a dependency relationship was set up when we# associated them with their articles just now.session.flush()print a1, a1.keywordsprint a2, a2.keywordsprint k_tutorial, k_tutorial.articlesprint k_cool, k_cool.articlesprint k_unfinished, k_unfinished.articles
Again, the code pretty much speaks for itself. If you pass the association table as the second parameter to mapping(), and SQLAlchemy sees that it‘s in the correct format (it has only two columns, each of which is a foreign key to one of the tables involved in the relation), it will automatically set up a many-to-many relationship for you.
One thing you may be interested in is the handy metadata.create_all() method. It will automatically figure out the dependency relationships between your tables, and create them in the proper order. It will also detect if the tables have already been created, and not try to re-create them a second time.
If you want to hold more data in the association object (for example, maybe you want to record the exact date and time when the article was tagged with any given keyword), it‘s slightly more complicated. The SQLAlchemy documentation has a good explanation of the process at the bottom of the Data Mapping page. That whole page, in fact, is well worth reading, since there are several other features of data mapping that I glossed over or left out entirely, in order to keep the size of this tutorial manageable. And once you‘ve grasped those concepts, you can move on to the Advanced Data Mapping section, which covers subjects like mapping a table onto itself (useful for tracking manager/subordinate relationships in an Employees table, for example).
New in 0.2: The create_all() function is new. Notice also that you need to explicitly save() the newly-created Article objects so that session.flush() will flush them to the database.
篇14:十七、PythonSQLAlchemy
Hopefully this has given you a taste of what using SQLAlchemy feels like. The examples in this tutorial should be enough to give you a head start in writing your own applications. When you need more advanced features beyond what we‘ve covered here, check out the extensive SQLAlchemy documentation. Just about everything you‘ll need is there; and if you still don‘t find what you‘re looking for, join the SQLAlchemy mailing list and ask!
篇15:十七、PythonSQLAlchemy
Remember how, in the joindemo.py tutorial, one of the comments said:
# There‘s a better way to do this, but we haven‘t gotten there yet
Well, now‘s the time to look at the better way to handle one-to-many and many-to-many relations. Copy the following into “mapper2.py”:
from sqlalchemy import *db = create_engine(‘sqlite:///joindemo.db‘)db.echo = Truemetadata = BoundMetaData(db)users = Table(‘users‘, metadata, autoload=True)emails = Table(‘emails‘, metadata, autoload=True)session = create_session# Let‘s give our User and Email classes a little more smartsclass User(object): def __init__(self, name=None, age=None, password=None): self.name = name self.age = age self.password = password def __repr__(self): return self.nameclass Email(object): def __init__(self, address=None): self.address = address def __repr__(self): return self.address# Here we look at several alternate ways to do the same thing. Try# running this program multiple times, enabling a different one of# these code blocks each time.# Let‘s look at several ways to do one-to-many relationships.# We create the Email mapper first...emailmapper = mapper(Email, emails)# ... so that we can use it in the User mapperusermapper = mapper(User, users, properties={ ‘emails‘: relation(emailmapper), # Here‘s where the magic happens})mary = session.query(User).get_by(name=‘Mary‘)print mary.emails# If we try to create multiple mappers associated with a single data# class, we have to specify which one is the “primary” mapper associated# with the class. Since we‘re demonstrating how to create one-to-many# relationships in multiple different ways, it‘s simplest to just clear# all the mappers and start over.clear_mappers()# We could also use the data class instead of the mapper as the parameter# to relation()emailmapper = mapper(Email, emails)usermapper = mapper(User, users, properties={ ‘emails‘: relation(Email),})mary = session.query(User).get_by(name=‘Mary‘)print mary.emailsclear_mappers()# In fact, we don‘t really need to keep a reference to the mapper object# around at all. Under most circumstances, we can just throw away the# object returned by mapper(). SQLAlchemy keeps track of a data class‘s# primary mapper behind the scenes, so we don‘t need to hold a reference# to it.mapper(Email, emails)mapper(User, users, properties={ ‘emails‘: relation(Email),})mary = session.query(User).get_by(name=‘Mary‘)print mary.emailsclear_mappers()# Notice that the order in which you create the mappers can be important.# If you want to call relation(), you need to pass it a class that‘s# already been mapped to a database table, or else SQLAlchemy won‘t be# able to figure out which table the ForeignKey relationships should refer# to. (Remember: “In the face of ambiguity, refuse the temptation to guess.”)try: usermapper = mapper(User, users, properties={ ‘emails‘: relation(Email), })except exceptions.InvalidRequestError: print “Ignoring the deliberately-provoked error and moving on...”clear_mappers()# What if we also want a “user” property on the Email class? Here‘s one# way to do it.emailmapper = mapper(Email, emails) # Save the mapper, to use it laterusermapper = mapper(User, users, properties={ ‘emails‘: relation(Email),})# Now that the User mapper has been created, we can use it in a call# to relation()emailmapper.add_property(‘user‘, relation(User))john = session.query(User).get_by(name=‘John‘)print john.emailscarl_address = session.query(Email).get_by(address=‘carl@nospam.net‘)print carl_address.userclear_mappers()# There‘s a handy “backref” feature that will do the above for youemailmapper = mapper(Email, emails)usermapper = mapper(User, users, properties={ ‘emails‘: relation(Email, backref=‘user‘),})# No need to call add_property(), it‘s already been donejohn = session.query(User).get_by(name=‘John‘)print john.emailscarl_address = session.query(Email).get_by(address=‘carl@nospam.net‘)print carl_address.userclear_mappers()# Order doesn‘t actually matter when you use backref: you can create the# “one” side of the one-to-many relationship first, or the “many” side of# the one-to-many relationship first, whichever way seems more natural# to you.usermapper = mapper(User, users)emailmapper = mapper(Email, emails, properties={ ‘user‘: relation(User, backref=‘emails‘),})john = session.query(User).get_by(name=‘John‘)print john.emailscarl_address = session.query(Email).get_by(address=‘carl@nospam.net‘)print carl_address.userclear_mappers()# If you‘ve created a relation(), you can now use object references# instead of object ID‘s to manage the relationship, and it all works# just like you‘d expect it to work.emailmapper = mapper(Email, emails)usermapper = mapper(User, users, properties={ ‘emails‘: relation(Email, backref=‘user‘),})harry = User(name=‘Harry‘, age=47)em1 = Email(‘harry@nowhere.com‘)em2 = Email(‘harry@example.org‘)em1.user = harry # Properly updates the harry.emails propertyharry.emails.append(em2) # Properly sets em2.user# Let‘s prove that harry.emails and em2.user were properly setprint em2.userprint harry.emailssession.save(harry)session.flush()clear_mappers()# Finally, let‘s demonstrate some other clever featuresemailmapper = mapper(Email, emails)usermapper = mapper(User, users, properties={ ‘emails‘: relation(Email, backref=‘user‘),})# If a relation has been defined, then get_by and select_by calls# can do the correct joins automaticallyprint session.query(User).get_by(address=‘mary@example.com‘)print session.query(Email).select_by(age=42)# This will only work if the column you‘re looking for is *not*# present in the “original” class, but is present in one of its# relations. For example, the following does *not* do a join to the# User table, but gets the user_id value from the Email table. Notice# the difference in the SQL that‘s printed.print session.query(Email).select_by(user_id=2)
Most of this is pretty self-explanatory. The relation() function is where all the magic happens. As you can see from the above example, it creates a property on the data class which will act like a list or a single object, as appropriate. When you‘re in the User class, reading the emails property, you‘re looking from the “one” side to the “many” side of the one-to-many relationship, so the property acts like a list. When you‘re in the Email class and reading the user property, you‘re looking from the “many” side to the “one” side of the relationship, so the property acts like a reference to a single object. The property has both getter and setter methods, so you can run code like “em1.user = harry” and the correct things will happen.
A quick note on the get_by() and select_by() functions we‘re using above. These are shorthand functions that take keyword arguments referring to the columns in the table we‘re selecting from. This lets you write “get_by(name=‘Mary‘)” instead of “selectfirst(users.c.name==‘Mary‘)”. If you specify multiple keyword arguments, they‘ll be joined together with AND. However, keep in mind a pretty severe limitation of the get_by() and select_by() functions, that comes directly from their use of Python keyword arguments. Keyword arguments are always in the form. “name=value”, which means that if you want to do anything other than an equality comparison, you need to use the full column name. E.g., “get_by(age<=39)” won‘t work; you need to do “selectfirst(users.c.name <= 39)” instead.
One final note: notice that in the penultimate example, when we created a new user Harry with two email addresses, we didn‘t call em1.save() or em2.save(). And yet both Email instances were also saved. This demonstrates how SQLAlchemy tracks object dependencies for you. The newly-created User instance held two references to Email instances in its emails property; therefore, the Emailinstances were dependent on their “parent” object (the User instance) and needed to be written out to the database at the same time. Notice also that the User object was created first, then the two dependent Email objects. That‘s because they needed to know the userid for Harry‘s newly-created database row, in order to set their own userid values properly. SQLAlchemy is very smart about dependency tracking, and will generally figure out the right order in which to do things. And if you have a very complicated example that SQLAlchemy can‘t figure out correctly, the author of SQLAlchemy considers that a bug and asks that you report it on the SQLAlchemy mailing list.
New in 0.2: As in the selectdemo.py example, we call select() functions on the Session object instead of on the mapper, via session.query(DataClass). That means that we often don‘t need to keep a reference to the mapper around, and makes it possible to simply throw away the results of the mapper() function call. It also means that the assign_mapper() function is less useful, soassign_mapper() isn‘t covered in this version of the tutorial.
篇16: 十七散文
十七散文
天空明净蔚蓝,夏的脚步悄无声息,阳光透过树缝照射在地面留下斑驳的影子,十七的影子。十七,是多现实的数字,是你独立的过渡。而此时的你在做什么?
十七,是个很青涩的年纪,也许你在上高中,也许你在谈恋爱,也许正是叛逆期。家里亲戚常说:每过一个年纪,我们都羡慕着,此刻总是怀念着正处于那年纪的人儿,羡慕她们还有年轻的机会。而我想送她们一句话,十七已过,我们无法挽回岁月的流逝,但你们还有爱的勇气、为偶像尖叫的权利、一颗易感动的`心,能为一切爱和美的事物落泪。
满眼青翠的绿意,润了眼睛丰盈了内心,掠过指尖的清风,吹起了绿。转眼十七快过了,似乎自己并未做过太多上进的事,十七的自己究竟做什么去了?
十七的自己,天天迷茫的游走在校园,无所事事,不知该做些什么。常常沉浸在自己的思绪里,无法自拔,常回忆曾经多少说好要永远在一起的人,早已被时光抛弃;多少感情,剩下的只能是背影,却细致得心碎也无悔。问自己,那些说好的约定,和诺言去哪儿了。放小长假或寒、暑假时,一个人四处流浪,看城市风景、看古镇典雅、看风俗民情……行走在途中会出现各种状况,奇葩事儿无处不有,但并没有影响我在路上的心情和念头。一路走,一路笑,一路感概,一路拍下美好瞬间。晚上,坐在窗边吹着风,望着星辰和它聊聊童年,聊聊现在,从亲情,到友情,到爱情,到天微微亮,简单的话题,我们却听到了彼此最温暖的故事,它们或感动,或忧伤,或温馨,但每一个都是藏在心里的秘密花园。我想,该入眠了。
天亮了,起身之前看了下长街上来往的车辆,路旁的灯光盏盏熄灭。忽觉得自己似乎“颓废”太久了,是时候努力了,以后便不再常熬夜罢。对,是时候努力了,再过几个月就成年了,不再是十七了,再过2年多就毕业找工作了,该为以后的人生好好想想了,为自己不做社会的累赘,不做啃老族,为对得起自己而努力,明天是新生,晚安!
篇17:十六后十七作文
十六后十七作文
我说花开花落,缘起缘灭,此时的偎依相伴,多了些韵味,我想经得住寂寞,接下来的青春路途洒下你们的身影,我不曾孤单。宿命论,抱歉,我还不敢相信,谋事在人成事在我,我不信命。青涩的感觉,陌生的面孔,有些招架不住,我有些慌乱,不知道未来的变数。一路走来,我可能太过顺利,本就未全力淋漓,未来的颜色,渐渐被我打乱,又重新上色,然后又被打乱,我也不知道自己究竟的样子,
我不知道十年后的未来的样子,我想我会习惯炎凉,我会冲淡杂念,我会在多年后的一个周末,喝着茶陪着一位老朋友,聊着青春,然后大家相视一笑,感叹现在的.我的天真,我还没有想到很多事,很多我要做但还有完成的事,我想多年后的我又会看淡很多事,还会忘记当初的很多事,也会慢慢不愿记起这些青春往事,我不确定能不能很坦然的说着笑着,聊着今天的烦心事,一年来,我体会到大喜到大悲,我从被喜欢但后来的被厌恶,我多次想过放弃,一切推倒,重新来过,这次,我算是如愿了,完全是个全新的世界,老一辈的离开,新一届的到来。
自尊心在作怪,我早已把自己看的最高,眼中的自己无论什么都是最好,才符合自己,我说环境太顺给了我炫耀的资本,而后的我再次回归平凡,我有些不知所措。微笑相依相伴,安之坦然若素,不恨时光渐渐,只看那些的年少轻狂,不愿将就。
十六后十七!我经历了很多,路过了完美,回归到平凡,现在的我更愿意尝试去慢慢接受。
篇18:十七星座200字作文
十七星座200字作文
白羊勇敢冲向前方,
金牛勤恳踏实坚强,
双子机智活力变幻,
巨蟹敏感极端柔软,
狮子光芒闪耀八方,
处女干净纯洁完满,
天秤娴雅迷人眼光,
天蝎神秘激情欲望,
射手乐观能吃会玩,
摩羯刚毅坚韧不拔,
水瓶自由奇思妙想,
双鱼眼泪稀里哗啦,
天马流星拳打冥王,
天龙庐山能治眼瞎,
白鸟冰河圣衣保暖,
仙女他哥不死凤凰,
横冲直撞有我天才樱木闪亮登场,
猩猩灌篮有我赤木刚宪…
六年级:灵影
【十七作文】相关文章:
1.十七作文550字
2.医学翻译词汇十七
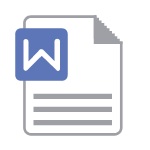





文档为doc格式